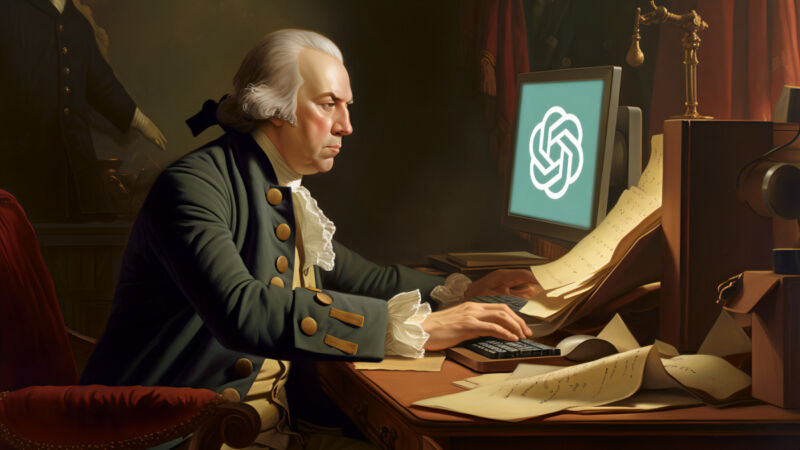
If you feed America's most important legal document—the US Constitution—into a tool designed to detect text written by AI models like ChatGPT, it will tell you that the document was almost certainly written by AI. But unless James Madison was a time traveler, that can't be the case. Why do AI writing detection tools give false positives? We spoke to several experts—and the creator of AI writing detector GPTZero—to find out.
Among news stories of overzealous professors flunking an entire class due to the suspicion of AI writing tool use and kids falsely accused of using ChatGPT, generative AI has education in a tizzy. Some think it represents an existential crisis. Teachers relying on educational methods developed over the past century have been scrambling for ways to keep the status quo—the tradition of relying on the essay as a tool to gauge student mastery of a topic.
As tempting as it is to rely on AI tools to detect AI-generated writing, evidence so far has shown that they are not reliable. Due to false positives, AI writing detectors such as GPTZero, ZeroGPT, and OpenAI's Text Classifier cannot be trusted to detect text composed by large language models (LLMs) like ChatGPT.
-
A viral screenshot from April 2023 showing GPTZero saying, "Your text is likely to be written entirely by AI" when fed part of the US Constitution.Ars Technica
-
When fed part of the US Constitution, ZeroGPT says, "Your text is AI/GPT Generated."Ars Technica
-
When fed part of the US Constitution, OpenAI's Text Classifier says, "The classifier considers the text to be unclear if it is AI-generated."Ars Technica
If you feed GPTZero a section of the US Constitution, it says the text is "likely to be written entirely by AI." Several times over the past six months, screenshots of other AI detectors showing similar results have gone viral on social media, inspiring confusion and plenty of jokes about the founding fathers being robots. It turns out the same thing happens with selections from The Bible, which also show up as being AI-generated.
To explain why these tools make such obvious mistakes (and otherwise often return false positives), we first need to understand how they work.
Understanding the concepts behind AI detection
Different AI writing detectors use slightly different methods of detection but with a similar premise: There's an AI model that has been trained on a large body of text (consisting of millions of writing examples) and a set of surmised rules that determine whether the writing is more likely to be human- or AI-generated.
For example, at the heart of GPTZero is a neural network trained on "a large, diverse corpus of human-written and AI-generated text, with a focus on English prose," according to the service's FAQ. Next, the system uses properties like "perplexity" and burstiness" to evaluate the text and make its classification.
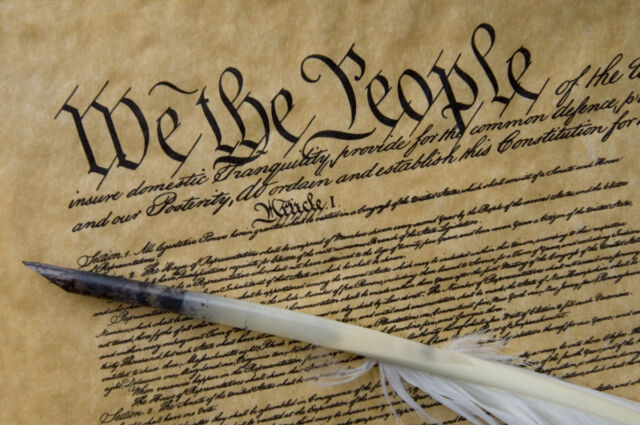
In machine learning, perplexity is a measurement of how much a piece of text deviates from what an AI model has learned during its training. As Dr. Margaret Mitchell of AI company Hugging Face told Ars, "Perplexity is a function of 'how surprising is this language based on what I've seen?'"
So the thinking behind measuring perplexity is that when they're writing text, AI models like ChatGPT will naturally reach for what they know best, which comes from their training data. The closer the output is to the training data, the lower the perplexity rating. Humans are much more chaotic writers—or at least that's the theory—but humans can write with low perplexity, too, especially when imitating a formal style used in law or certain types of academic writing. Also, many of the phrases we use are surprisingly common.
Let's say we're guessing the next word in the phrase "I'd like a cup of _____." Most people would fill in the blank with "water," "coffee," or "tea." A language model trained on a lot of English text would do the same because those phrases occur frequently in English writing. The perplexity of any of those three results would be quite low because the prediction is fairly certain.
Promoted Comments